Engineering
Test clocks: How we made it easier to test Stripe Billing integrations
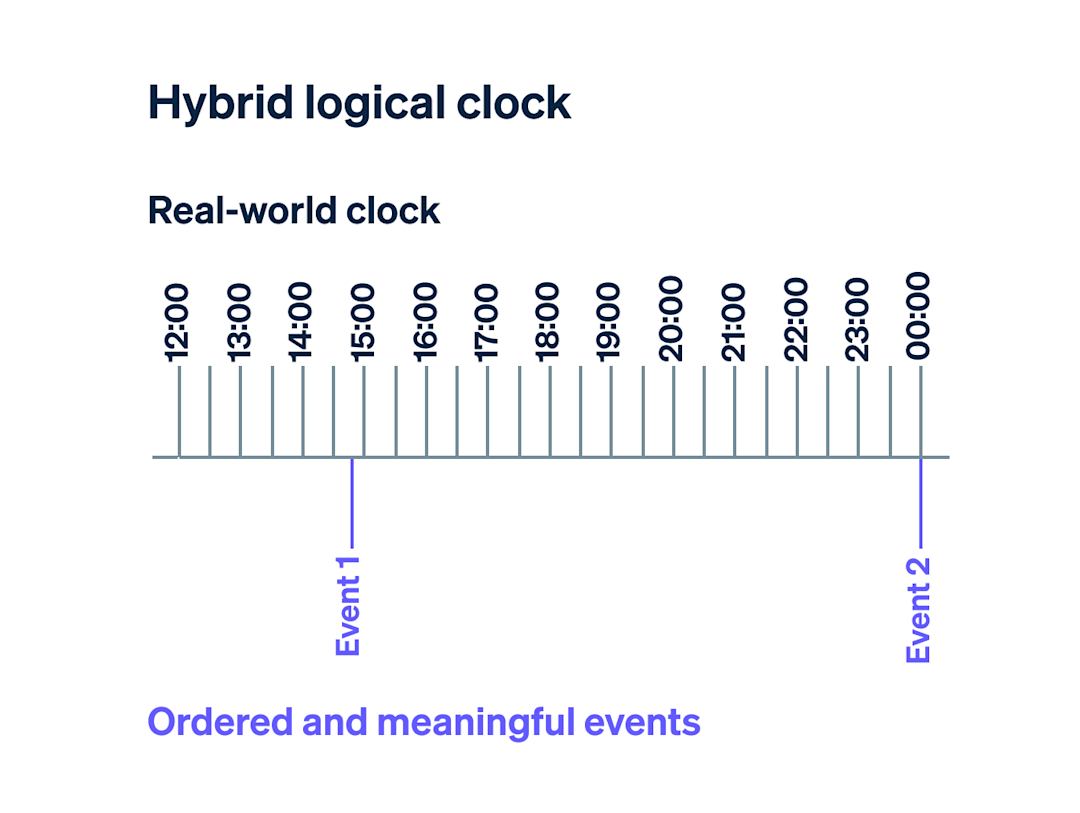
Stripe Billing allows businesses to manage customer relationships with recurring payments, usage triggers, and other customizable features.
We love the web, and care deeply about beautiful code, APIs, and documentation. This belief is as true today as it was when we first wrote this line on our website when we were ten people. Our engineering blog captures how, what, and why we code at Stripe.
Stripe Billing allows businesses to manage customer relationships with recurring payments, usage triggers, and other customizable features.
This blog discusses the technical details of how we built Shepherd and how we are expanding the capabilities of Chronon to meet Stripe’s scale.
In this blog post, we’ll share technical details on how we built this state-of-the-art money movement tracking system, and describe how teams at Stripe interact with the data quality metrics that underlie our payment processing network.
You can now configure payment method settings from the Dashboard—no code required. We call this integration path dynamic payment methods.
Radar is Stripe’s fraud prevention solution. This post covers what makes Radar so powerful and some of the key decisions we’ve made—and lessons we’ve learned—over the almost seven years we’ve been building it.
Delivering a good user experience without compromising the authoring experience required us to develop an authoring format that enables writers to express interactivity and simple page logic without mixing code and content.
While developing Markdoc, we learned how to balance interactivity, customization, and authoring productivity while undertaking a major overhaul of our documentation platform.
On Sunday, March 6, we migrated Stripe’s largest JavaScript codebase from Flow to TypeScript. In a single pull request, we converted more than 3.7 million lines of code. The next day, hundreds of engineers came in to start writing TypeScript for their projects.
Stripe's continuous integration (CI) system is responsible for orchestrating build pipelines and executing the tens of thousands of test suites that our engineers depend on to validate their changes. We lean on a combination of open-source technologies and novel engineering to deliver a CI system that is performant, secure, and delivers a delightful developer experience.
Sorbet has been a crucial part of Stripe’s growth in recent years. Today, Sorbet runs over our entire Ruby codebase, currently amounting to over 15 million lines of code spread across 150,000 Ruby files. Sorbet increases productivity and fosters a collective engineering culture. Here’s why we love Sorbet.
Abstracting away the complexity of payments has driven the evolution of our APIs over the last decade. This post provides the context and conceptual frameworks behind our API design—and the milestones that led to the PaymentIntents API.
We set out to build a globe that inspires a sense of awe, invites people to explore, and conceals details for discovery. Along the way, we evaluated existing tools, designed our own solution, solved four interesting technical challenges, and improved the way we collaborate. Here’s what we learned.
Last May, Stripe launched our remote engineering hub, a virtual office coequal with our physical engineering offices in San Francisco, Seattle, Dublin, and Singapore. It’s now the backbone of a new working model for the whole company. Here is what we’ve learned.
Stripe enables businesses in many countries worldwide to onboard easily so they can accept payments as quickly as possible. Stripe’s scale makes our platform a common target for payments fraud and cybercrime, so we’ve built a deep understanding of the patterns bad actors use. We take these threats seriously because they harm both our users and our ecosystem; every fraudulent transaction we circumvent keeps anyone impacted from having a bad day.
Building and testing a Stripe integration can require frequent switching between the terminal, your code editor, and the Dashboard. Today, we’re excited to launch the Stripe command-line interface (CLI). It lets you interact with Stripe right from the terminal and makes it easier to build, test, and manage your integration.
Color contrast is an important aspect of accessibility. Good contrast makes it easier for people with visual impairments to use products, and helps in imperfect conditions like low-light environments or older screens. With this in mind, we recently updated the colors in our user interfaces to be more accessible.
Stripe launched in Singapore in 2016. Since then, we’ve seen strong traction, and are proud to work with some of the fastest-growing companies in the region, including Grab, Mobike, and Carousell. Today, we’re increasing our investment: we’re very excited to announce that Singapore is joining Seattle, Dublin, and San Francisco to become Stripe’s fourth global engineering hub.
Logging is one of the oldest and most ubiquitous patterns in computing. We’ve found using a slight augmentation to traditional logging immensely useful at Stripe—an idea that we call canonical log lines. It’s a simple technique: in addition to their normal log traces, requests also emit one long log line at the end that includes many of their key characteristics. Having that data colocated in single information-dense lines makes queries and aggregations over it faster to write, and faster to run.
DNS is a critical piece of infrastructure used to facilitate communication across networks. DNS issues can quickly lead to crippling, widespread outages, and you could find yourself in a real bind. Here's how we monitor our DNS systems and how we used an array of tools to investigate and fix an unexpected spike in DNS errors that we encountered recently.
Stripe uses machine learning to respond to our users’ complex, real-world problems. Machine learning powers Radar to block fraud, and Billing to retry failed charges on the network. Our machine learning infrastructure scores hundreds of millions of predictions across many machine learning models. Over time, the volume, quality of data, and number of signals have grown enormously. Here we discuss Railyard and our lessons on building and operating machine learning infrastructure.
Stripe has engineering hubs in San Francisco, Seattle, Dublin, and Singapore. We are establishing a fifth hub that is less traditional but no less important: Remote. We are doing this to situate product development closer to our customers, improve our ability to tap the 99.74% of talented engineers living outside the metro areas of our first four hubs, and further our mission of increasing the GDP of the internet.