Engineering
How Stripe’s document databases supported 99.999% uptime with zero-downtime data migrations
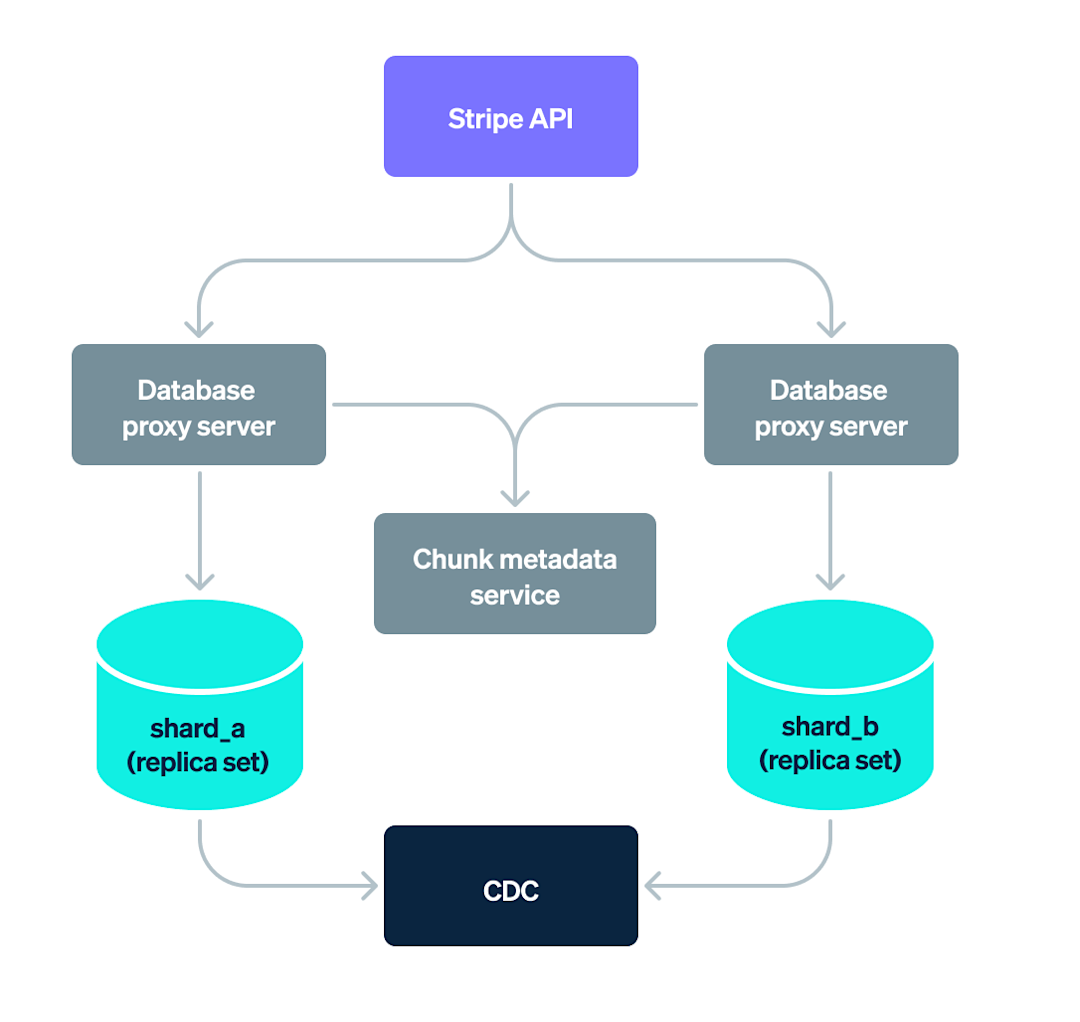
In this blog post we’ll share an overview of Stripe’s database infrastructure and discuss the design and application of the Data Movement Platform.