Product
The untapped opportunity at the bottom of your customer funnel
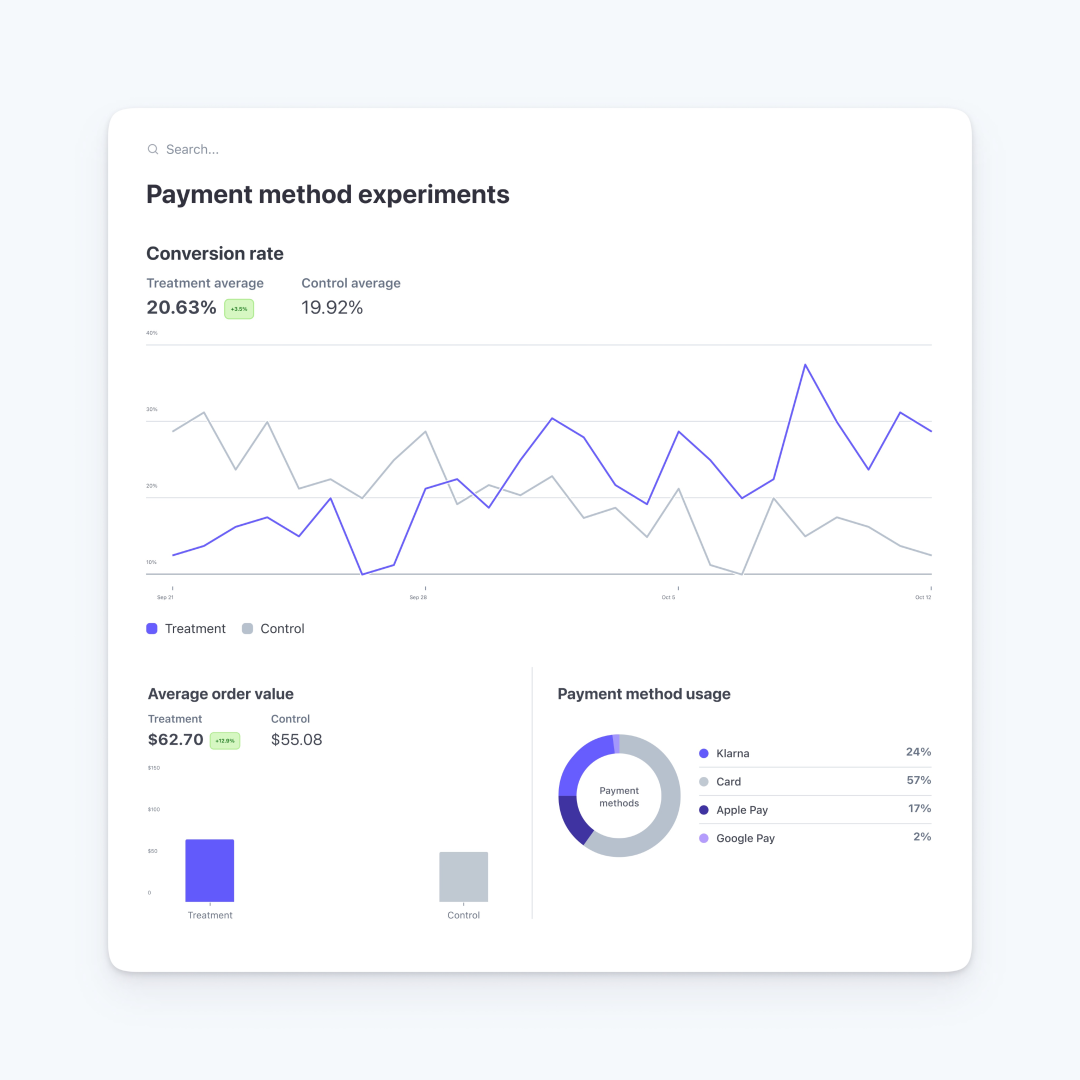
Businesses spend vast resources attracting customers, only to lose sales at the very last stage of the customer journey—the checkout. This week we’re releasing new features to our optimized checkout suite to help businesses change that.