This report offers a comprehensive overview of the state of online fraud. We analyzed data from 2019–2022, including billions of attempted payments across millions of businesses on Stripe, and worked with Milltown Partners (in partnership with Focaldata) to survey more than 2,500 business leaders in 9 markets around the world (Australia, Canada, France, Germany, Japan, the Netherlands, Singapore, the United Kingdom, and the United States).
By combining our own Stripe analysis with these survey results, we’re able to identify the biggest fraud trends in the past year—such as the increase in product-related disputes in 2020 and that recurring revenue businesses are particularly concerned about the financial impacts of fraud. We also highlight how you can successfully adapt to these fraud trends with tips throughout the report based on the data we uncovered. We end this report with four overarching best practices based on our predictions for where we see the fraud industry going.
We are categorizing this report into four sections:
- Why fraud has increased
- How fraud differs by region, country, and company size
- The business impact of fraud
- Our predictions for the fraud industry
Executive summary
- According to our survey, 64% of global business leaders say that since the onset of the pandemic it has become harder for their business to fight fraud. We believe this is due, in part, to an increase in types of fraud and overall fraud volume.
- At the start of the pandemic, we observed a temporary 156% increase in product-related disputes, such as “product not received” and “product not acceptable” dispute codes. We hypothesize that customers were requesting chargebacks after sellers were taking weeks, or even months, to fulfill orders due to supply chain disruptions.
- We also saw that 40% more businesses experienced attempted card testing attacks. Thousands of new ecommerce businesses were created during the pandemic, and we believe this growth created new opportunities for fraudulent actors.
- Businesses around the world experienced increases in fraud; however, businesses in Latin America were—and continue to be—particularly susceptible to fraud attacks. We observed that businesses in Latin America had a 97% higher fraud rate compared to those in North America and a 222% higher fraud rate than businesses in the Asia-Pacific region. This is due to a variety of region-specific factors, such as a locally run payments infrastructure.
- Recurring revenue businesses—specifically B2C companies—struggled the most with fraud. More than 75% of B2C subscription businesses reported that their manual review load increased and that they had to divert more resources to fight fraud in the last year. We believe that these consumer-facing businesses have more brand awareness, meaning their products are easier to resell. As a result, fraudulent actors are more likely to target them.
- The business impact of fraud goes beyond financial losses. Our Stripe analysis found that the more fraud a business tries to prevent, the more likely they are to block legitimate charges as well—reducing their payment conversion rates. In an effort to reduce these false positives, businesses can manually review flagged payments, but this adds additional operational overhead.
- We predict that businesses will adapt to these trends in four ways: 1) Interventions, such as 3DS, will play a bigger role; 2) Richer sources of data will help businesses make faster, more accurate decisions; 3) Issuers and businesses will collaborate more to streamline disputes and reduce false declines; and 4) Consumer payment preferences will continue to shift, changing the fraud landscape.
Why fraud has increased
COVID-19 ushered in a historic wave of ecommerce growth. Businesses on Stripe processed more than $640 billion in payments in 2021, up 60% from the prior year. These payments came from a rapidly growing group of businesses: 1,400 new companies joined Stripe each day last year. This growth—especially in new businesses—created more opportunities for fraudulent actors.
Many were starting businesses for the first time and lacked the tools or resources to deal with fraud, or they were more focused on setting up their business and becoming profitable than creating a fraud prevention strategy. But these challenges weren’t just reserved for new businesses—even established businesses found it harder to prevent fraud due to more complex types of fraud or higher volumes of fraud compared to pre-pandemic times.
At the same time, fraudulent actors continue to become more sophisticated. They find new ways to target businesses, often organizing into groups and connecting with other fraudulent actors to share “best practices.”
As more shoppers shop online at our stores, the volume of fraud payments has increased. It is hard to manually review all transactions, so we focus on a select few since there [are] not enough [resources].
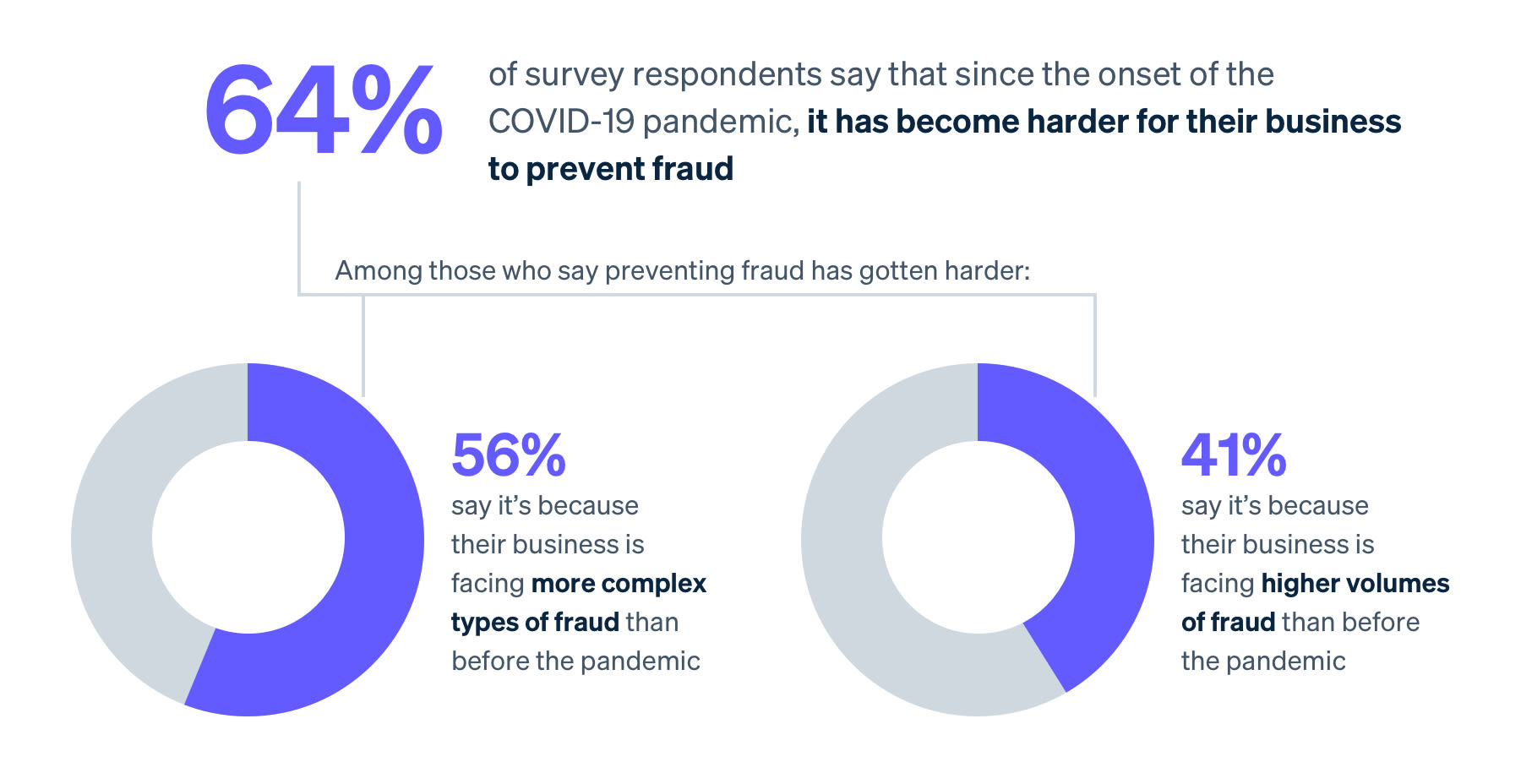
Product-related disputes doubled in 2020 compared to 2019
From March 2020-May 2020, our Stripe analysis found that payments were more than twice as likely to result in non-fraudulent-related reason codes, such as “product not received” and “product not acceptable” disputes, compared to 2019. We hypothesize that customers were requesting more chargebacks after sellers were taking weeks, or even months, to fulfill orders due to supply chain disruptions.
Latin America seemingly experienced the lowest rates of product-related disputes, but we believe this finding is due to issuer behavior. In Mexico, disputes are seven times as likely to be reported without a reason code as all countries combined, and in Brazil, disputes are 50% more likely to be reported as fraud.
Best practices for preventing product-related disputes:
-
Make your return policy clear, transparent, and reasonable. For example, start the return window when a customer receives the item instead of when the item is shipped.
-
Add your company name directly in your credit card descriptor.
-
Establish a formal dispute process.
-
Notify customers before processing their payment. For subscription companies, make sure customers receive at least one reminder of their upcoming payment.
-
For ecommerce businesses, require a customer’s signature when delivering their order.
Attempted card testing attacks targeted 40% more businesses
Card testing occurs when someone tries to determine whether stolen card information is active so that they can use it to make purchases. A fraudulent actor may do this by purchasing stolen credit card information and then attempting to validate or make purchases with those cards to determine which cards are still valid.
During the first year of the pandemic, we saw a 40% spike in the proportion of businesses experiencing attempted card testing attacks. This trend applied to both new and established businesses; however, new businesses (those that had signed up on Stripe within 90 days) made up a bigger share than usual of card-tested companies.
Card testing attacks can negatively impact businesses in a number of ways. The influx of transactions due to a card testing attack can lead to higher payment processing costs and the risk of downtime (if a business can’t handle the increase in traffic, their website can crash). In addition, successful card testing attacks damage the global financial ecosystem. Businesses are more likely to process payments from stolen cards, ultimately resulting in more disputes. Because of the risk to the financial ecosystem, businesses may be penalized by issuers and card networks for allowing card testing attacks.
A separate Stripe analysis from November 2021 found that charities are particularly impacted by card testing attacks: 11% of all card testing attacks we observed were targeted at charities. Why? Many charities allow donors (or in this case, fraudulent actors) to choose a very small donation amount, such as $1.00 or $5.00. Small transactions are less likely to be noticed by the real cardholder on a statement. In addition, charities are more likely to have smaller fraud teams and lack the resources to block transactions. Not only do charities (and any card testing business) lose the money, they are also penalized by banks for allowing these card attacks to happen.
Best practices for preventing card testing attacks:
-
Optimize your integration with your payments provider. Many payments providers will apply different controls to mitigate a card testing attack, but the success of those controls depends on the quality of your integration and the signals you send to the provider. In general, the more data your integration provides, the more successful card testing prevention can be.
-
Keep your API keys safe. Your secret API key can be used to make any API call on behalf of your account, such as creating charges or performing refunds. Treat your secret API key as you would any other password and only grant access to those who need it.
-
Enable CAPTCHA in your checkout flow to differentiate between legitimate customers and card testing bots.
-
Set rate limits to control the amount of incoming and outgoing traffic. For example, if card testers validate cards by attaching them to new customers, you could limit the number of new customers that come from a single IP address in one day.
-
Consider requiring customers to log in to their account to make a payment.
How fraud differs by region, country, and company size
The importance of fighting fraud is universal: More than 90% of leaders we surveyed say that preventing ecommerce fraud is important to their business. There are, however, subtle differences in fraud activity based on company industry and location, suggesting a complex picture.
Fraud by region and country
Stripe has the most payment volume data for businesses in North America, so we will use North America as the baseline for other regions in this section’s analysis.
All online businesses have to manage fraud; however, our Stripe analysis showed that businesses in Latin America were particularly susceptible to increasing fraud rates.
Our data showed that Latin America had the highest card fraud rates in the world during our studied timeframe: 97% higher than North America and 222% higher than the Asia-Pacific region. Locally run payments infrastructure and less frequent credit card usage mean that fraud models used by banks can be weaker than in other regions. Rules also tend to favor cardholders in the dispute process, causing businesses to be especially vulnerable to fraud. In addition to these local factors, the market is increasingly moving online (we saw a 518% increase in new businesses started on Stripe in Latin America in 2021), creating even more opportunities for fraudulent actors to attack.
Businesses in Europe, the Middle East, and Africa had substantially lower fraud rates compared to North America, which likely reflects the impact of Strong Customer Authentication (SCA) regulations mandating that businesses add two-factor authentication to their checkout flow.
There was also considerable variation among countries. For example, France had nearly double the fraud rate of Germany, while Singapore experienced half the fraud rate of the Asia-Pacific region as a whole. This variation in fraud across countries can make it even more difficult for global businesses to fight fraud. As a result, there is never a one-size-fits-all approach to fraud management.
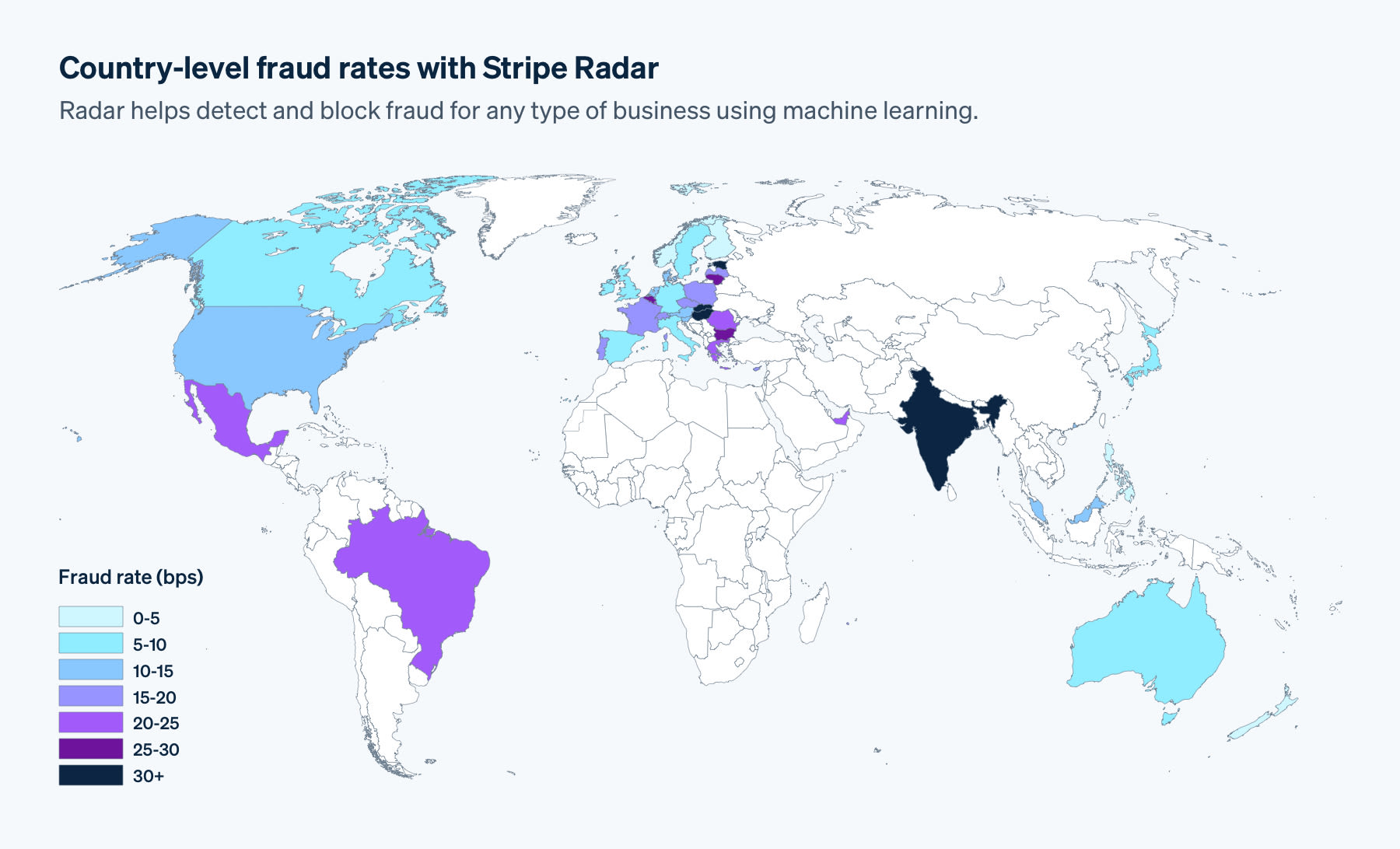
If you have the capacity and the bandwidth, we recommend analyzing your customers’ behaviors, market trends, and regulations in each country in which you operate to better understand the most likely fraud attacks and vectors you might experience. However, as businesses scale, this complexity can quickly become too much to manage, underscoring the importance of leveraging a sophisticated, automated fraud tool.
Fraud by company size and business model
Business leaders perceive the risk of fraud differently depending on company size and business model. For example, our survey showed that fraud prevention gets more important with scale and, unsurprisingly, larger businesses have more resources to invest in that fraud prevention strategy compared to smaller companies. However, resources alone don’t prevent fraud. According to our survey, business leaders with large fraud teams were more likely to face operational challenges managing fraud and are more likely to report higher fraud losses.
These trends may point to opportunities for smaller businesses: Growing businesses may choose to develop an in-depth fraud strategy now, when they are smaller, to get ahead of the problem. However, diverting time and resources to fight fraud may come at the expense of business growth, and smaller businesses should carefully consider the trade-offs.
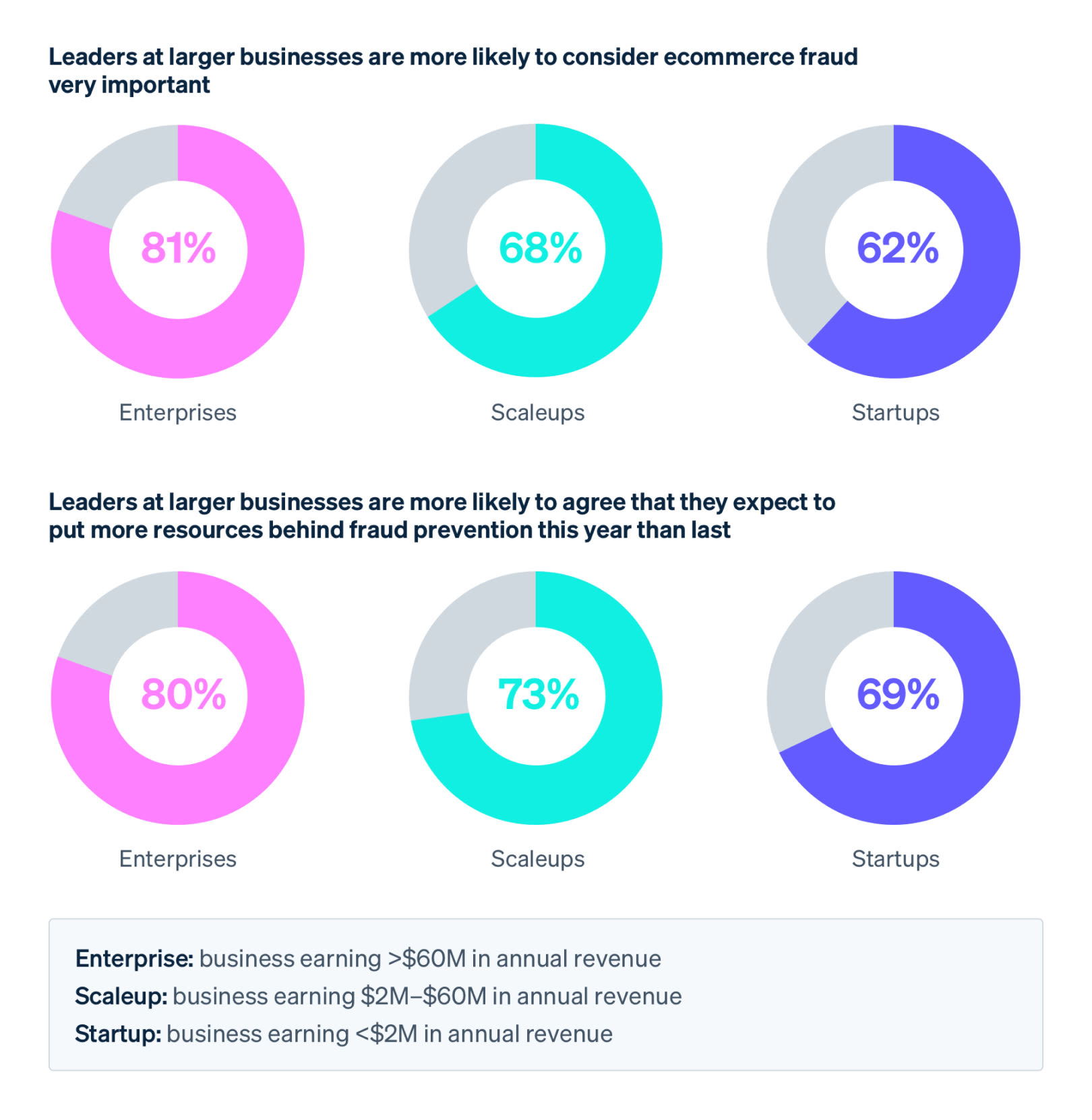
We also analyzed our survey results based on business model, categorizing companies into the following:
- Software-as-a-service (SaaS)
- B2C subscriptions
- Marketplaces and platforms
- Ecommerce
We found that recurring revenue businesses were the most concerned about the financial impact of fraud. Compared to the other business models we surveyed, fraud leaders at recurring revenue companies were more worried about losing money to fraud and more likely to think they lost a higher proportion of their revenue to fraud in 2021 compared to pre-pandemic times. These worries may be a result of their business model: Because they generate revenue on a set schedule (such as monthly or quarterly) and because they have seen their fraud rates increase in the past year, they are more likely to think that trend will only continue as their business grows.
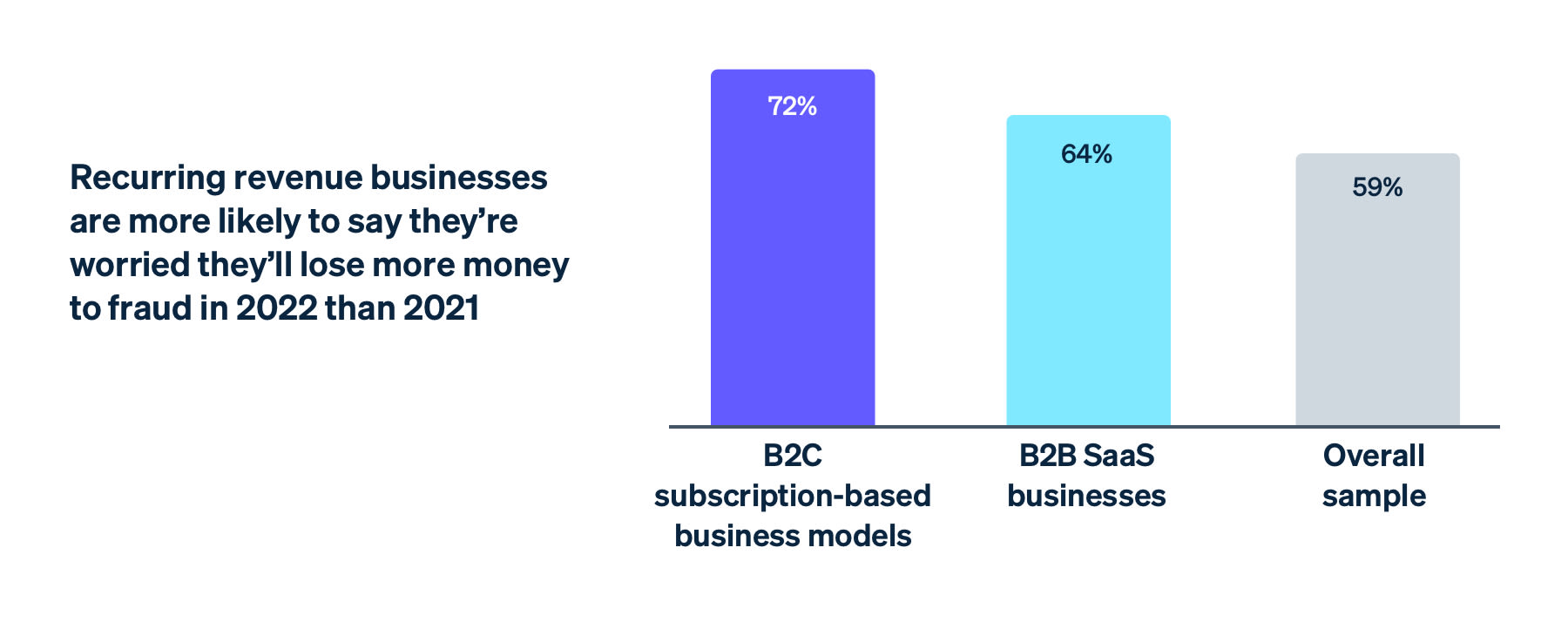
In particular, B2C subscription businesses struggled more with the operational burden of fraud. They were more likely to report that their manual review cases increased in 2021, that they have diverted more resources to fight fraud, and that they’ve had to delay investments or expansion plans in order to manage fraud.
We hypothesize that B2C businesses experienced more fraud because they are more likely to be household brands, making it easier for fraudulent actors to resell the stolen goods or services (such as buying a digital subscription with a stolen credit card, then selling it for a lower price).
The business impact of fraud
Fraud is expensive. In fact, 59% of survey respondents expect their business to lose more revenue to fraud this year than last.
Businesses lose money to both fraudulent disputes and trying to prevent that fraud. For example, if your business loses a dispute, you are responsible for paying more than just the original transaction amount. Fraud often leads to chargeback fees (the cost associated with the bank reversing the card payment) and higher network fees from disputes.
However, our survey found that the business impact of fraud goes beyond just financial losses. Many businesses have to grow their fraud team or divert product or engineering resources to manage operational overhead, shifting valuable resources away from their core product.
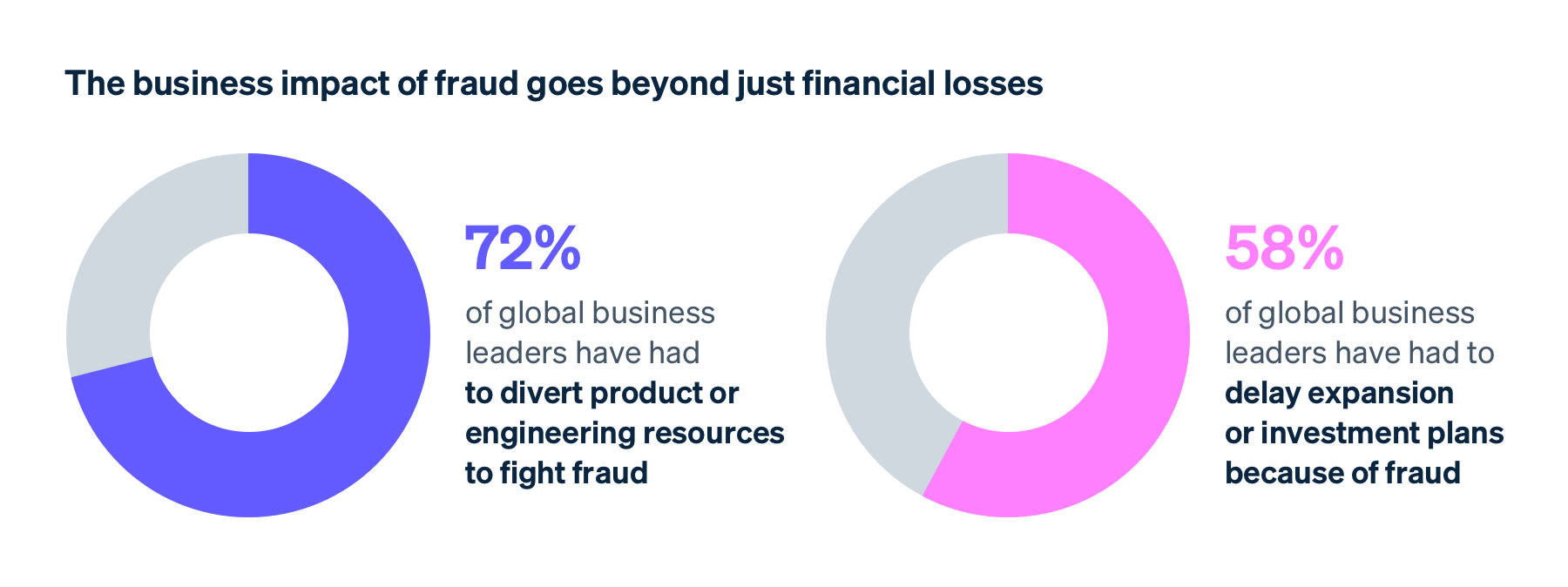
Lower payment conversion rates
In our Stripe analysis, we found that the more fraud a business tries to prevent, the more likely they are to block legitimate charges as well.
False positives, or false declines, are when a legitimate customer tries to make a purchase but is prevented from doing so. False declines can cause the business to take both a gross profit and reputational hit. In fact, 33% of consumers said they wouldn’t shop again at a business after a false decline.
Even a single fraud issue [can] cause a lot of trouble and potentially makes us miss a legitimate buyer due to additional security reviews.
There is a trade-off between preventing more disputes and reducing the number of legitimate customers blocked. When you prevent more fraud, you’ll increase the number of good customers blocked. On the other hand, reducing the number of good customers erroneously blocked often increases the likelihood of more true fraud slipping through the cracks. This trade-off also depends on your fraud solution: You will always have to manage this trade-off if your fraud solution is static and you don’t invest in ongoing resources to improve it. On the other hand, if your fraud solution’s models continually adapt and change based on fraud vectors, this trade-off can be less of a challenge.
Given the trade-off between preventing disputes and blocking legitimate payments, businesses can select the threshold at which to block payments in order to maximize profit. This profit-maximizing point is where the difference between fraud costs prevented and good profit blocked is largest.
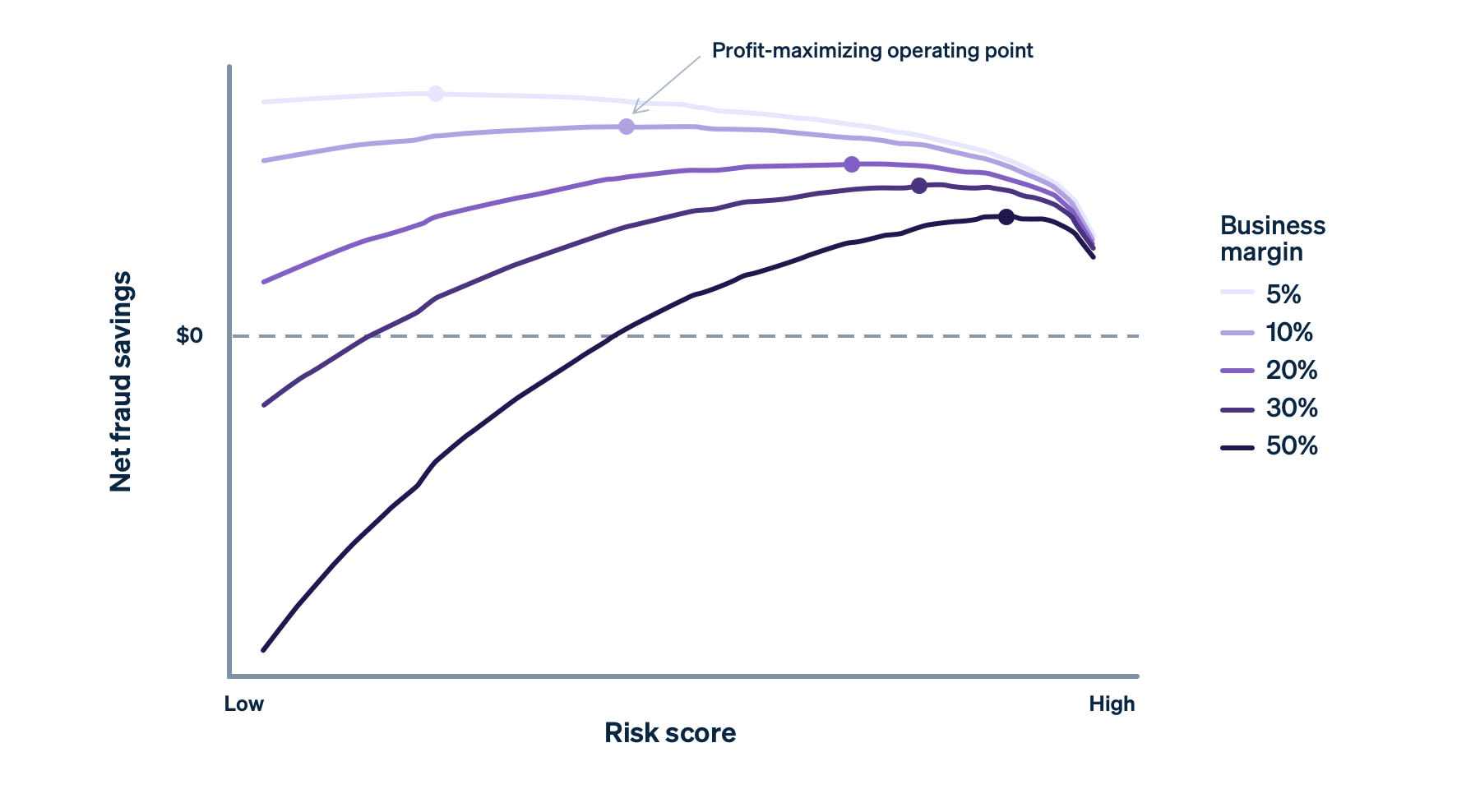
Risk score is the threshold at which to block transactions using Radar (the default settings block transactions when they exceed a risk score of 75).
Net fraud savings is the result of the total fraud costs prevented minus the legitimate profit blocked.
Profit-maximizing operating point is the exact point at which a business has maximized net fraud savings, optimizing between blocking fraudulent transactions and blocking good transactions.
How to read this graph: As the risk threshold increases along the x-axis, there is a higher likelihood of a transaction being fraudulent. The higher the risk threshold, the fewer transactions you block. As you block more transactions, your net fraud savings increases—but you are also more likely to block legitimate transactions as well.
The tradeoff between preventing fraud and blocking legitimate transactions depends on the per-transaction margin. For example, businesses with high-margin (50%) transactions along the dark purple line in the graph may be more likely to allow more transactions and have a higher risk threshold because each individual, legitimate transaction is so much more valuable (compared to a lower margin business, for example
Businesses need to manage this trade-off based on their margins, growth profile, and other factors. If a business’s margins are small—for example, if you sell food online—the cost of a fraudulent transaction might need to be offset with hundreds of good transactions—making each false negative very expensive. Businesses with this profile may lean toward casting a wide net when attempting to stop potential fraud. On the other hand, if a business’s margins are high—say for a SaaS business—the reverse is true. The lost revenue from one legitimate blocked customer may outweigh the cost of increased fraud. It’s also important to note that businesses can choose how they optimize their fraud rates up to a certain point—if fraud reaches certain levels, card networks will impose fees and fines.
Operational overhead
In an effort to reduce false positives, businesses can manually review flagged payments to confirm whether they are truly fraudulent. This is quite labor intensive; businesses need a team of fraud analysts to assess risk based on a variety of factors, such as transaction details and customer history.
It’s really frustrating because it means that I have to divert resources to accommodate it or else I feel that the situation will get out of hand.
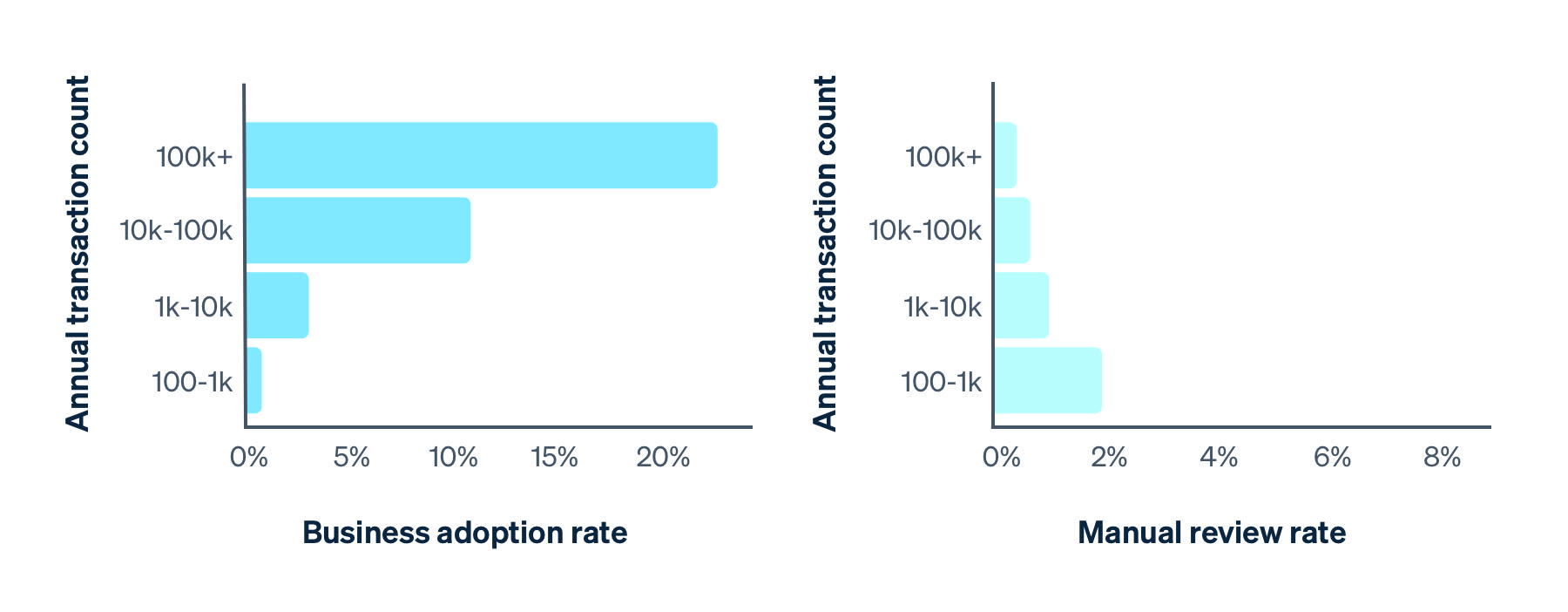
Proportion of active, eligible Stripe businesses that use manual reviews (business adoption rate) and the average proportion of transactions manually reviewed (manual review rate) by number of transactions in the last year (listed numbers are upper bounds of buckets)
We found that larger companies are more likely to adopt manual reviews, but the larger they are, the smaller the fraction of transactions they review. For example, more than 20% of businesses who had more than 100K transactions in the last year used manual reviews, but they reviewed less than 1% of their total transactions. Large businesses have the resources to manually review transactions, but they save those manual reviews for higher-stake transactions.
Recommendations to reduce operational overhead:
-
For smaller businesses without dedicated fraud teams, a chargeback guarantee solution (where a third party guarantees to cover chargeback costs) can be particularly helpful.
-
For medium-sized to large ecommerce businesses, a machine learning solution can help fight fraud at scale, without requiring extra engineering resources.
-
Large enterprises often use a handful of point solutions (like specific tools to support CAPTCHA or card scanning) in conjunction with fraud software or as inputs into their own fraud models.
Our predictions for the fraud industry
Fraud constantly evolves over time, and 2021 was no exception. In fact, fraudulent actors became even more sophisticated last year, targeting online businesses in new ways. We’ve covered a number of the challenges in this report, but what does this mean for your business? We believe businesses should adapt to the current fraud landscape in four ways:
1. Interventions, such as 3DS, will play a bigger role
Interventions allow you to more confidently block or allow transactions when you think they’re suspicious by issuing a “challenge” to customers (like asking them to enter a one-time code that is sent via text).
Interventions can take many forms, including:
- 3DS, which requires customers to complete two-factor authentication to make a payment. It is the main card authentication method used to meet Strong Customer Authentication (SCA) requirements in Europe and a key mechanism for businesses to request exemptions to SCA.
- Identity verifications, such as asking customers to scan a government ID to verify their identity.
- Card scans to confirm that the customer has their physical card in their possession at the time of the transaction.
- CAPTCHA tools that require website visitors to solve a simple puzzle, such as transcribing a series of numbers or letters from a distorted image.
Interventions are already gaining in popularity. We analyzed the activity of one specific intervention, 3DS, among Stripe businesses in 2021 and found that 3DS adoption increased across the board, with the strongest gains outside of North America. As expected, European businesses experienced the biggest increase in 3DS adoption (this was a result of SCA requirements being fully enforced in almost all eligible European countries last year). SCA-like regulation is also growing in popularity outside of Europe, increasing the fastest in India.
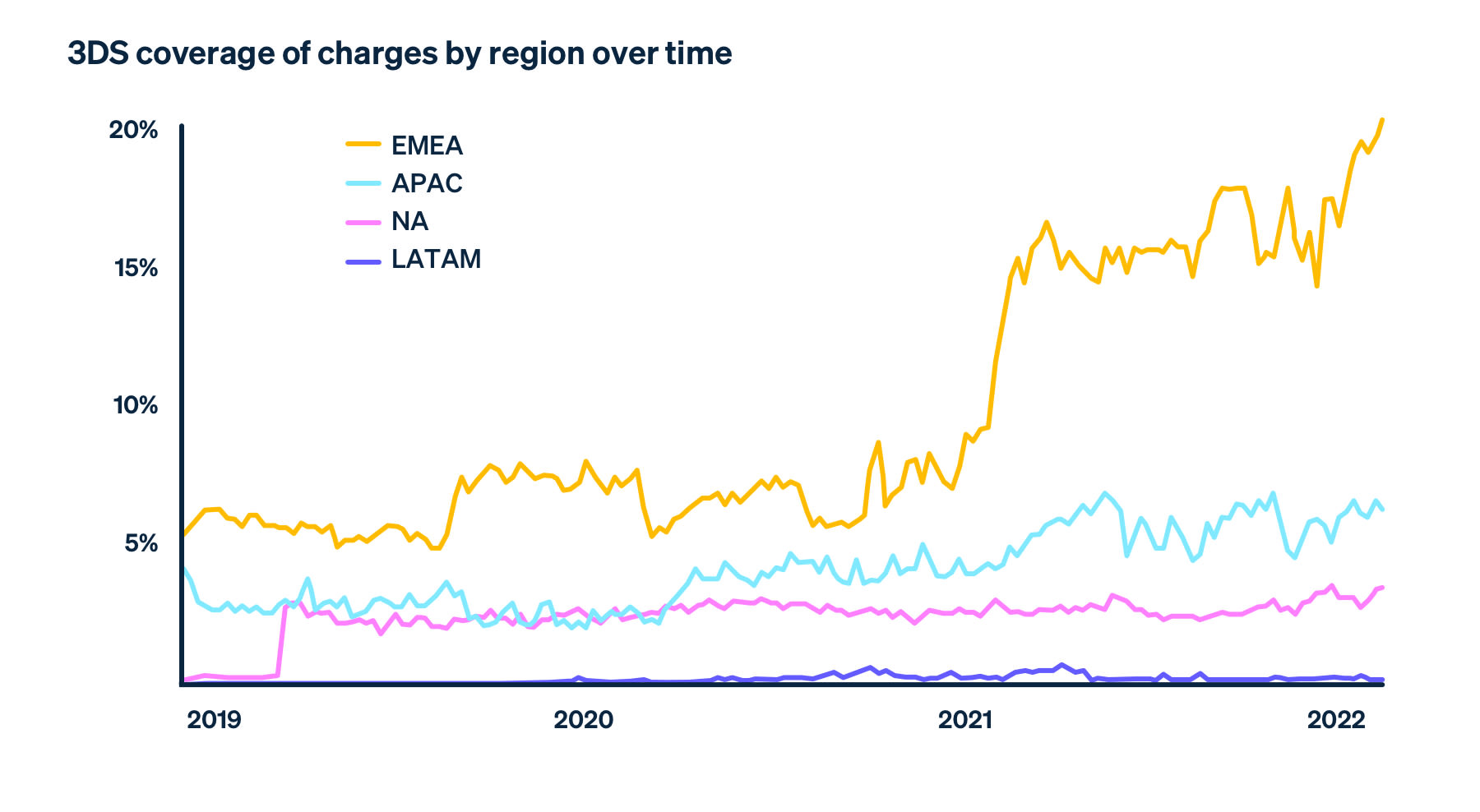
In one experiment, Stripe found that lowering the threshold at which 3DS is triggered resulted in a 74% decrease in the fraudulent dispute rate. In addition, compared to blocking charges outright, 3DS still allows the majority of payments to be successful (67% across all risk levels, 5% for elevated risk level). However, 3DS performance can vary across issuers.
Going forward, we expect the use of interventions to increase. Businesses will apply interventions to more of their transaction volume and use more diverse types of interventions, especially those that reduce the amount of friction in the checkout process.
Tips for using interventions:
-
Replace the transactions that you currently block with interventions to increase conversion and avoid blocking legitimate charges.
-
Interventions can introduce friction in the customer experience, which can negatively impact conversion. Carefully optimize and test how you want to trigger interventions to ensure you’re not adversely affecting legitimate customers.
-
Each intervention has a different pass rate and a different impact on reducing fraud. For example, while security keys are extremely effective at preventing fraudulent actors, they can dramatically hurt conversion. Choose the right intervention based on the riskiness of the action that your customer is performing and your risk and conversion tolerance.
-
Run interventions where they make the most logical sense in the user journey (such as requesting a physical card scan when a customer is adding their card details).
2. Richer sources of data will help businesses make faster, more accurate decisions
Fraud management used to be highly manual, requiring a team of analysts to review each and every transaction. Today, the majority of businesses use some level of machine learning models and automation to fight fraud at scale, in addition to manual reviews when necessary (this hybrid approach varies depending on industries and business models). Machine learning models learn how to discern legitimate transactions from those that are potentially fraudulent, and some can even train themselves, making them more scalable and efficient.
Machine learning models were once considered cutting-edge technology for fighting fraud, but they are now table stakes. In fact, machine learning capabilities on their own are no longer enough to mitigate the ever-evolving risks of fraud. Our survey respondents agree: More than half of respondents, whose review process is mostly automated, said that the type and amount of fraud they face is evolving too rapidly for their business to keep up.
The opportunities for financial fraud have become more diverse and complex over time. We need to constantly adapt to new fraud patterns and opportunities.
We believe the next phase in the evolution of fraud management will focus on richer data to inform fraud models. The tools and technology to gather this information are available today, but they are often in siloed, disparate systems; businesses may have separate tools for identity verification and biometrics, for example. In the future, we predict that businesses will be able to leverage better technology and integrations to consolidate this information in one place, providing a holistic approach to make fraud models more efficient.
By looking at relevant data from across the customer journey—including behavioral, biometrics, and enriched third-party data related to phone numbers, email addresses, the untapped reservoir of issuer data, and even social networking platforms—businesses can achieve new levels of fraud detection accuracy.
While this level of data is very useful for improving fraud models, businesses must exercise caution when collecting and storing this information to ensure compliance with global data security and privacy laws.
3. Issuers and businesses will collaborate more to streamline disputes and reduce false declines
When a customer completes a purchase on your site, your payments provider takes the charge details and sends them through the card networks, like Visa, Mastercard, or China UnionPay, to the issuing bank (the customer’s bank) as a payment request. The issuing banks, such as Chase, Citi, and Barclays, are the ultimate decision-makers when approving or declining a transaction during the authorization phase. They calculate the fraud risk based on the signals they receive during authorization, which are fairly limited.
Businesses, on the other hand, have rich customer and transaction data, such as a customer’s email and billing addresses. Combining this data with the information the issuer already has can lead to a higher percentage of transactions being accepted.
Improved authorization and fraud rates are mutually beneficial—the issuing bank can reduce fraud losses, save on operational costs, and increase transaction volume by reducing the number of customer inquiries on false declines. At the same time, businesses enjoy higher payment conversion rates and improved customer retention. However, most businesses still don’t share this data with issuers, leading to an information asymmetry that contributes to the $443 billion of false declines in 2021.
We now see a shift, with issuers investing in building enhanced authorization APIs, such as Capital One’s Enhanced Decisioning Data API and Amex’s Enhanced Authorization API. Large businesses, for which every percentage point uplift in authorization manifests in millions of dollars, also understand the importance of data partnerships and are beginning to invest in integrating with issuers. Yet, there is a gap for the millions of other businesses that don’t have the technical capacity or significant payments volume to justify the ROI of bespoke issuer integrations. For these businesses, we expect financial partners such as Stripe and other payments providers to help facilitate this exchange by leveraging their scale and built-in issuer partnerships.
4. Consumer payment preferences will continue to shift, changing the fraud landscape
Payment methods like buy now, pay later, digital wallets, and crypto cards without card numbers printed on the card (like the Gemini Credit Card) are on the rise. Buy now, pay later services have particularly increased in adoption: More than half of US customers have used a buy now, pay later service, and it was the fastest-growing payment method in 2020 in India and the UK.
All payment methods used for online transactions carry some level of fraud risk, and non-card methods are no different. For example, payment methods like buy now, pay later can be more susceptible to new account fraud (where fraudulent actors create new identities to open fraudulent accounts during the onboarding flow, which may be poorly protected) and account takeovers (where a malicious third party gains access to a customer’s account credentials and uses their payment information to make fraudulent purchases).
However, businesses can mitigate these risks by focusing on fraud prevention strategies earlier in the customer lifecycle. Rather than focusing on the transaction itself, businesses can screen for fraudulent activity earlier in the customer journey to make an assessment before the customer (or fraudulent actor) even makes a purchase. For example, businesses should confirm a customer’s identity during onboarding, check for duplicate accounts, and enforce identity verification measures (such as two-factor authentication) at login.
How Stripe can help
Stripe is a fully integrated suite of payments products that powers payments for online and in-person retailers, subscriptions businesses, software platforms and marketplaces, and everything in between. From beating fraud to verifying identities, millions of businesses use Stripe to:
Optimize the checkout experience
- Collect more relevant information during checkout: Asking customers to provide more relevant information at checkout will help you better verify their legitimacy. For example, make sure to collect the customer’s name and email address. This additional information can be passed to Stripe Radar, resulting in better machine learning detection of fraud and giving you more evidence to submit during a potential dispute.
- Explore other payment methods: The right set of payment methods can offer flexibility to customers and reduce the risk of fraud. Digital wallets, like Apple Pay or Google Pay, require additional customer verification (such as biometrics, SMS, or a passcode) to complete a payment, resulting in lower dispute rates. Similarly, most bank debits—where you pull funds directly from a customer’s bank account—require customers to agree to a mandate or to verify account ownership, adding an extra layer of security and reducing the possibility of disputes.
Prevent fraud during checkout
- Leverage machine learning fraud detection: Rules-based fraud detection, operating on an “if x happens, then do y” logic, was never designed for modern internet businesses and can lead to lost revenue. Stripe Radar is powered by adaptive machine learning, with algorithms evaluating every transaction and assigning a risk score, then blocking or allowing transactions based on the risk of fraud. Radar’s algorithms adapt quickly to shifting fraud patterns and to your unique business.
- Prevent fraud and increase authorization through issuer partnerships: Stripe’s issuer partnerships share select risk data when possible to help issuers block fraudulent transactions while approving legitimate ones. Integrating with issuers creates value for both the cardholder and the business: Customers can shop more with greater confidence while businesses get more transactions approved without an increase in fraudulent disputes.
- Dynamically apply two-factor authentication: Stripe Checkout can handle European SCA requirements and dynamically apply authentication, such as 3DS, when required by the cardholder’s bank or when fraud is suspected. Stripe Checkout also supports the simplest method of PCI validation with a pre-filled SAQ A, and it triggers CAPTCHA only when we suspect card testing attacks, to prevent fraud.
Manage fraud with your team
- Create rules to customize fraud: Using Radar for Fraud Teams, you can create custom rules to manage how your business handles incoming payments, blocking any that you would consider suspicious or placing them in review. For example, you could lower the risk score required to trigger manual reviews or review large orders from first-time customers. Radar for Fraud Teams also provides risk insights into particular payments, allowing you to understand the most important factors contributing to a high risk score. You can use this information to create additional, more targeted rules.
- Manually review high-risk payments: Radar for Fraud Teams includes an additional review process that allows you to flag certain payments for review (although these payments are still processed and the credit card is charged). While Radar for Fraud Teams is commonly used by larger organizations, the ability to manually review payments is helpful, regardless of your company’s size (although smaller businesses have found manual reviews to be especially useful). Manually reviewing suspicious payments can help you take action more accurately, before a potential dispute occurs. For example, if you’re unsure about a payment when you’re reviewing it, you can contact the customer by phone or email. Or, if you suspect a payment is fraudulent, you can refund it.
Additional fraud prevention tips
- Access deeper insights on fraud trends: Stripe Sigma allows you to quickly analyze your Stripe data via predefined or custom SQL queries in the Stripe Dashboard. Answer your complex business questions, from understanding why customers dispute payments to what percentage of disputes you contest. You can also use Stripe Data Pipeline to send up-to-date Stripe data to your Snowflake or Amazon Redshift data warehouse. This allows you to easily combine your Stripe fraud risk scores with other fraud data to pull richer fraud reports.
- Verify global customers: Stripe Identity lets you programmatically confirm the identity of global users so you can reduce attacks from fraudulent actors with minimal friction for legitimate customers.
- Optimize conversion and recover more revenue: Stripe Card Image Verification helps reduce the number of erroneously blocked transactions. Instead of blocking potentially high-risk transactions, it gives users a chance to confirm they have the card they say they do by asking them to scan a picture of their card (launching in 2022).
To learn more about how Stripe Radar can help your business fight fraud, contact sales or sign up for an account.
Additional resources
Here are additional resources to help you manage fraud and protect your business:
Methodology
Stripe analyzed billions of attempted payments from millions of businesses from 2019-2021. Across those payments and businesses, we looked at disputes and their reasons, predictions from our machine learning models, 3DS usage, and businesses’ manual review activity. For country-level fraud rates, we excluded countries with fewer than 10,000 payments in 2021 from our analysis because they had too few transactions to reliably calculate fraud rates.
In early 2022, Stripe also worked with Milltown Partners (in partnership with their data provider, Focaldata) to survey more than 2,500 business leaders in 9 markets around the world (Australia, Canada, France, Germany, Japan, the Netherlands, Singapore, the UK, and the United States) who estimate their businesses make at least 10% of their revenue from online sales.